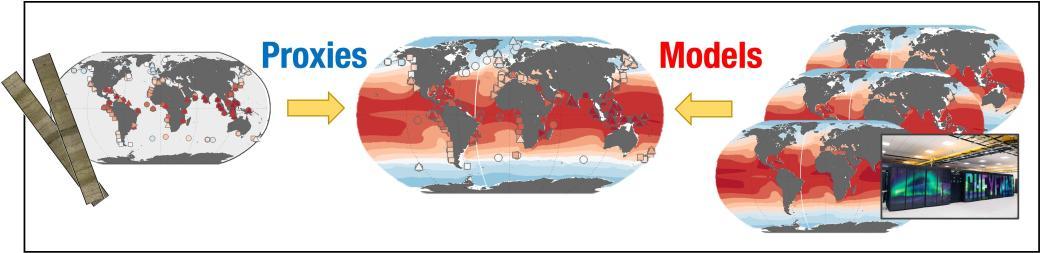
Lead supervisor: Matthew Osman, Geography
Co-supervisor: Marco A. Aquino Lopez, Geography; Francesco Muschitiello, Geography
Brief summary:
Newly compiled climate proxy measurements will be combined with climate insights from general circulation models using physics-based “statistical learning” methods to reconstruct targeted intervals of the recent geologic past and refine projections of future climate.
Importance of the area of research concerned:
With the pace of climate change accelerating, there is need to understand and prepare for the full spectrum of possible future climate impacts. Projections from climate model simulations provide us with such insight, but validation of these models and reduction of their uncertainties is challenged by the limited time span of modern observations. Study of Earth’s recent geologic past (ca. 3 Ma to present) offers the potential to refine the dynamical mechanisms of global climate states both significantly colder and warmer than present and, with the ongoing proliferation of new proxy measurements and paleoclimate modelling capabilities, we are now beginning to truly tap into the “big data” that Earth’s geologic past entails. Yet, with this exciting data deluge come new challenges in pairing together these data in physically sound, statistically grounded ways. The recent advent of offline ensemble paleo-data assimilation approaches (e.g., Tierney et al., 2020) has provided an elegant means of efficiently combining large and diverse paleoclimatic proxy and model datasets. However, statistical optimisation and application of these approaches remains a challenge.
Project summary :
This project will seek to develop physics-based “statistical learning” methods to reconstruct targeted warm and cold intervals of our recent geologic past and refine future model-based uncertainties, using models and climate proxy data. The first phase will develop statistical methods to automate the prescription of model- and proxy-based uncertainties and optimise the tuning parameters needed to refine assimilation-based reconstructions. After validating this framework across multiple background climate states, the student will then apply their framework in phase two to generate new monthly-resolved reconstructions of the Mid Pliocene Warm Period (ca. 3 Ma), Last Interglacial (ca. 127), and Last Glacial Maximum (ca. 21) intervals, to provide fully probabilistic “emergent constraints” on possible future climate impacts and large-scale climate reorganisations (e.g., tipping points).
What will the student do?:
The student will work to develop new, computationally tractable statistical learning algorithms applied to paleoclimate data assimilation and paleoclimate emergent constraint problems. To achieve project aims, the student will be expected to assist in compiling new and (or) revising pre-existing proxy datasets; in generating globally consistent proxy chronologies using state-of-the-art probabilistic dating software; in developing or refining Bayesian proxy system models; and in compiling, analysing and interpreting multi-model climate model output spanning past and future. The student will be expected to regularly share their research findings at domestic/international meetings, and to the public alike.
References - references should provide further reading about the project:
Hall et al. Progressing emergent constraints on future climate change. Nat. Clim. Change, 9, 269–278 (2019). https://doi.org/10.1038/s41558-019-0436-6
Kageyama et al. The PMIP4 contribution to CMIP6 – Part 1: Overview and over-arching analysis plan, Geosci. Model Dev., 11, 1033–1057 (2018). https://doi.org/10.5194/gmd-11-1033-2018
Tierney et al. Past climates inform our future. Science, 370, eaay3701 (2020). https://doi.org/10.1126/science.aay3701
Applying
You can find out about applying for this project on the Department of Geography page.